Using remote sensing for early detection of diseases and pests in agriculture
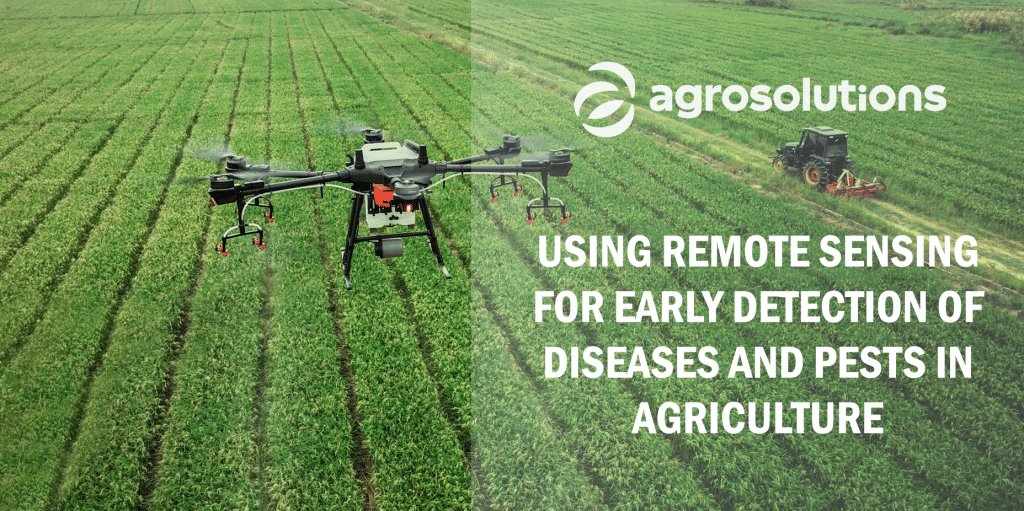
The damage caused by diseases and pests on French farms equates to an annual yield loss of 10 to 20%. [1] The most advanced control tools French farmers use are chemically synthesized products. These chemical processes are effective in reducing damage to farm yields however, without proper pest and disease detection systems, these chemical processes can be overused. Remote sensing technology is an effective new method of loss prevention which allows farmers to monitor and reduce losses due to pests and diseases all the while reducing working loads and time.
What is remote sensing?
Remote sensing is a tool that uses sensor-based spatial imaging technologies combined with the use of data processing algorithms as well as artificial intelligence.
The remote sensing process begins with the collection of data recovered by vectors[2], usually satellites or drones, equipped with high-resolution sensors. The sensors used can collect electromagnetic data in various frequency bands. The most common frequency bands used are visible light (RGB[3]) and infrared (RE, NIR, IR[4]). These frequency bands are effective as the RGB and infrared waves emitted by natural or artificial sources reflect off vegetation and can easily be detected by purpose-built sensors. Rendered images generated from sensor data are stored in databases that can be viewed via geographic information systems (GIS).
The advantage of spatial imaging is that it provides almost instantaneous information on an extensive area. The constellations of networked satellites, which are increasingly dense, receive image updates on average every 2 day. The data from the vectors can be input into specialty software and analyzed by a specialist, it can then be transformed into useful information and utilized for decision-making.
Remote sensing is an increasingly common tool in agriculture. For example, the possibility of obtaining the vegetative state of a crop in real time or the degree said crop is affected by disease is a considerable advantage and aids in management of the farm. The data acquired by spatial imaging makes it possible to prevent the effects of disease on a large scale and much earlier than with the human eye. The data also presents a degree of reliability often greater than common predictive decision-making tools.
Multinational satellite companies have understood the incredible market potential of precision agriculture. The European Space Agency was a leader in Earth Observation (EO) missions with Sentinel and Copernicus in 2015. Combined, these companies are continuously providing large amounts of satellite images that are publicly available around the world.
A “new eye” for farmers
The OenOMIP project, led by TerraNIS (a company specializing in the design of geoinformation services) and the Purpan Engineering School (INP), is interested in the detection of Golden Flavescence (a disease widespread in French vineyards) using space imagery gathered via drone vectors.
An image taken by drone may reflect the internal cellular composition of a leaf when viewed through a thermal sensor. The most commonly used indicators for the detection of biochemical parameters are the leaf area index (LAI[5]) and the rate of photosynthetic radiation (fAPAR[6]). The vine leaf absorbs and reflects specific wavelengths. The difference between symptomatic and asymptomatic leaves can be seen by comparing the reflectance emitted by each leaf (Figure 1). On symptomatic leaves, reflectance is more pronounced in the spectrum of the visible (RGB) and the Red Edge (at the limit of the visible). Its reflectance is, however, lower in the near infrared (NIR) band. Higher reflectance in blue and red frequencies indicates a chlorophyll deficiency. Reflected electromagnetic energy changes according to the grape variety. Once the disease develops, it can be detected through color changes in the leaves of diseased vines.
Figure 1 – Reflectance of the symptomatic leaves of Golden Flavescence (red) and asymptomatic leaves (green) from a plot of marselan (black grape variety) (a) and chardonnay (white grape variety) (b) vines.
Spatial imaging provides the ability to detect infections before symptoms are visible to the naked eye and more quickly than a field inspection. After the drone gathers data, the farmer can easily determine which grapevines are infected (Figure 2).
Figure 2 – Examples of drone images in false color in 2 vineyards (Gamay, Duras) in September 2015 and location of grapevines infected by the golden flavescence. For each vineyard, a comparison between the image obtained by remote sensing and the observation on the ground confirms the results.
The potential and limits of remote sensing tools
The main advantage of spatial imaging is that it gives access to a large amount of data almost instantaneously. These images offer information sometimes undetectable to the human eye, without mobilization of a workforce in the field.
Insecticides and fungicides can present a significant operational burden on farms. These plant protection products can have a negative impact on the environment if applied in an uncontrolled and excessive manner. Furthermore, some dangerous chemicals may affect non-targeted organisms. Among these organisms, the decline in key species populations can lead to rapid degradation of the ecosystem, thus negatively impacting the environment. To manage these financial and environmental risks, remote sensing coupled with an in-depth analysis of the situation can be a solution. It will help farmers optimize the application of products and spray solutions by selecting targeted areas and specific times to apply them to crops.
Reducing the use of these products and spray solutions can therefore be both an economic benefit to the farmer and beneficial to the health of the farm biosphere. However, remote sensing tools are not free of charge. Costs are incurred for either hiring a service, which ranges from 40 to 60€/ha, or purchasing equipment, which can range from 10000 to 20000€ for a drone with sensors[7]. The many existing services prove that the use of remote sensing has been profitable over several years.
Remote sensing remains a tool that requires human interpretation. The interpretation of satellite images requires time and technical knowledge. If we take the example of the tool used for the Golden Flavescence, it only indicates a change of reflectance on the vine leaf which could have been caused by other factors than this specific disease. It is therefore important to keep in mind that a trained workforce remains necessary in order to provide reliable advice on agronomic practices.
A solution to the third path of agriculture
Data analysis remains time-consuming and requires a certain level of specialization. The increasing capabilities of artificial intelligence applications/software can be a valuable tool in this task.
The database accumulated by the spatial observation of our environment will allow a greater understanding of agroecosystems and systemic interactions. Understanding the interactions of agricultural environments is the first step in applying context specific practices in order to minimize losses caused by pests and diseases.
Beyond the advantages of adapting practices to specific situations and the comprehension of processed data, this solution is particularly relevant as part of the deployment of the 3rd path of agriculture. The 3rd path of agriculture is attempting to offer consumers an agriculture that responds to societal issues. It is based on three fundamental pillars: the farmer (intuitu personæ), the environment and food.
Remote sensing responds to the first pillar by saving farmers time and financial savings in optimizing plant protection products. It also contributes to the environmental pillar by making it possible to reduce the application of chemical solutions through a discriminated application. Finally, the food pillar is also supported by imaging tools as they reduce the loss of yield due to diseases and pests, while guaranteeing the sanitary quality of the products.
Remote sensing will most likely be one of the major solutions to meet consumer expectations of today and tomorrow.
Sources:
- Johanna Leslie Albetis de la Cruz. 2018. Potential of multispectral images acquired by drone in the detection of areas infected by the Flavescence dorée of the vine. Université Toulouse 3 Paul Sabatier: https://tel.archives-ouvertes.fr/tel-01907912/document
- European Spatial Agency: https://www.esa.int/
- Jacques Lerin, Erick Rivault. 1988. Yield losses associated with 2 successive pests (Ceuthorrhynchus napi Gyll. and Meligethes aeneus F.) on winter rape (Bienvenu variety). Laboratoire de zoologie, INRA: https://hal.archives-ouvertes.fr/hal-00885096/document
- Benoit Hervieu. 2019. The digital eye on the crop. INRAE: https://www.inrae.fr/actualites/dossier-teledetection-oeil-numerique
- Benoit de Solan, Mathilde Closset. The potentials and limits of drones in agriculture. Arvalis: https://www.arvalis-infos.fr/the-potentialites-and-limits-of-drones-in-agriculture-@/view-15555-arvarticle.html
- Pierre Criado. 2017. A CUMA drone is profitable. ENTRAID: https://www.entraid.com/articles/un-drone-en-cuma-rentable#:~:text=Le%20pack%20Airinov%20comprenant%201,euros%20au%20prix%20catalogue%20classique
- The diagnosis by vine. Scanopy: https://www.scanopy.fr/solutions-cartographie-vigne/diagnostic-parcelle-vigne/
- ADJUST, CONTROL AND MODULATE YOUR NITROGEN DOSES TO THE INTRA-ARCELLARY VARIABILITY OF YOUR PLOTS. BeApi: https://beapi.coop/be-api-solutions/api-azote-plus/
[1]Source: (Lerin and Rivault. 1988)
[2] Machines carrying imaging sensors: drones, planes, satellites, etc.
[3] Frequency bands: RGB = Red, Green, Blue
[4] Frequency bands: RE = Red Edge; NIR = Near Infrared; IR = Infrared
[5]LAI = Leaf area index
[6] fAPAR = fraction of Active Radiation from Photosynthesis
[7]Source: ENTRAID, BeApi, Scanopy